


To plot the residuals, use the command plot(lmTemp$residuals). Otherwise means that maybe there is a hidden pattern that the linear model is not considering.
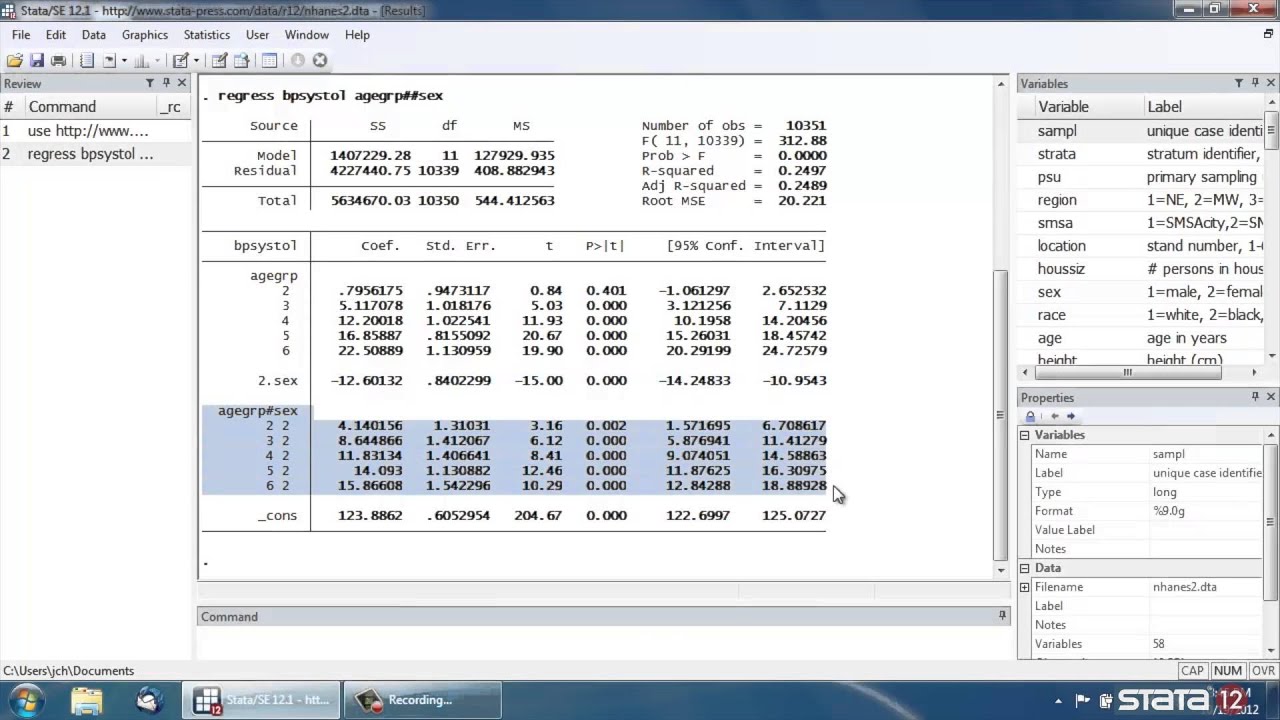

Ideally, when you plot the residuals, they should look random. Residual standard error: 42.66 on 8 degrees of freedom With the command summary(lmHeight) you can see detailed information on the model’s performance and coefficients. The lm command takes the variables in the format:
Linear regression veusz download#
Download the data to an object called ageandheight and then create the linear regression in the third line. The data to use for this tutorial can be downloaded here. To know more about importing data to R, you can take this DataCamp course. In the next example, use this command to calculate the height based on the age of the child.įirst, import the library readxl to read Microsoft Excel files, it can be any kind of format, as long R can read it. In general, for every month older the child is, his or her height will increase with “b”.Ī linear regression can be calculated in R with the command lm. The slope measures the change of height with respect to the age in months. Newborn babies with zero months are not zero centimeters necessarily this is the function of the intercept. With the same example, “a” or the intercept, is the value from where you start measuring. In this case, “a” and “b” are called the intercept and the slope respectively. In this particular example, you can calculate the height of a child if you know her age: In the previous example, it is clear that there is a relationship between the age of children and their height. This means that you can fit a line between the two (or more variables). In this case, linear regression assumes that there exists a linear relationship between the response variable and the explanatory variables. Not every problem can be solved with the same algorithm. It’s even predicted it’s still going to be the used in year 2118! Creating a Linear Regression in R Even though it is not as sophisticated as other algorithms like artificial neural networks or random forests, according to a survey made by KD Nuggets, regression was the algorithm most used by data scientists in 20. It’s simple, and it has survived for hundreds of years. This is precisely what makes linear regression so popular. Linear regression is one of the most basic statistical models out there, its results can be interpreted by almost everyone, and it has been around since the 19th century. You make this kind of relationships in your head all the time, for example when you calculate the age of a child based on her height, you are assuming the older she is, the taller she will be. In reality, a relationship may exist between the dependent variable and multiple independent variables.A linear regression is a statistical model that analyzes the relationship between a response variable (often called y) and one or more variables and their interactions (often called x or explanatory variables).
Linear regression veusz full#
Linear regression veusz how to#
In this post, you’ll see how to perform a linear regression in Python using statsmodels.
